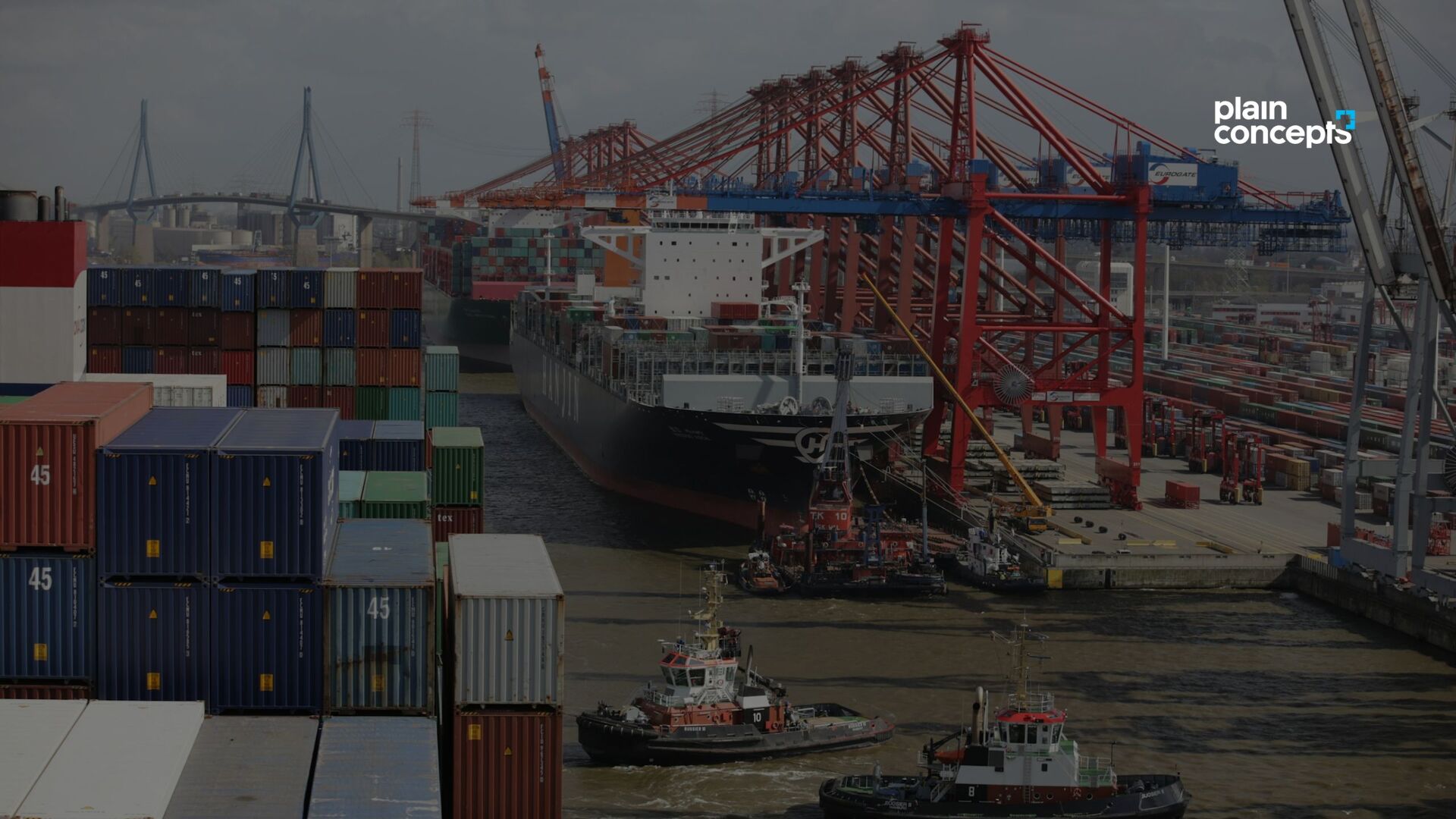
GenAI: the big driver of the Supply Chain
Generative AI is becoming one of the great allies for improving processes and customer experience in many industries. The supply chain sector is no exception, as we are facing a situation of accelerated efficiency.
However, many companies are encountering problems when it comes to implementing them. We have compiled the keys to achieving the best results and how to ensure that your company gets the most out of this technology.
How is today’s Supply Chain market?
Since 2020, the global economy has entered a new paradigm marked by much faster changes, the irruption of more disruptive technologies, more interconnected impacts, and more demanding users.
This is why COOs and supply chain managers must monitor this high-risk climate frontier and bear the brunt of disruptions. Many companies have chosen to increase the resilience of supply chains by diversifying their operations across multiple countries and suppliers. However, this has, in many cases, resulted in reduced efficiency.
With generative AI, companies can benefit from this help to overcome their technical maturity and accelerate their path to an autonomous chain. This technology can be used not only to analyze and interpret large amounts of data but also to create new scenarios, generate innovative solutions, and eliminate friction in real-time. This translates into end-to-end visibility and frees up human time for higher-order work.
The use of AI in this sector is not new, as many cases of automation and data-driven insights have already been seen. However, with generative AI, new processes can be designed, future demands can be predicted more accurately to alleviate external impacts, and routes can be identified more easily to facilitate and optimize carriers’ work.
In addition, with the combination of GenAI and traditional AI, the gap to autonomous supply chains can be closed. This level of creativity and adaptability is crucial for developing supply chains that can dynamically adjust to changing market conditions and operate with limited human intervention.
On the surface, all are advantages. However, EY research of 460 global supply chain and operations executives found that only 28% have achieved a supply chain with low human intervention and only 50% have achieved end-to-end visibility across the entire supply chain.
AI and Supply Chain
Companies in the industry recognize the potential of this technology and see it as a key capability to remain competitive in the future. According to EY, three-quarters plan to implement GenAI in their supply chains and 80% believe it can reinvent their supply chains and are prioritizing it.
However, in the last year, only 7% have completed the implementation of technology in their supply chain. The main reasons are:
- Concern and lack of understanding about the unique risks GenAI generates.
- Challenges of implementing this complex technology.
Industry leaders are finding that the most difficult challenge is making the leap from PoC to generative AI on a large scale. However, this presents a great opportunity to evolve and transform business models into autonomous and robust supply chains.
The pioneering companies that have already moved down this path are also the ones demonstrating the greatest success in results. In fact, they are the most ambitious in terms of implementing GenAI use cases in the coming months.
But to do so, integrating this technology is not just a matter of implementing advanced technology, but also requires a strategic perspective that encompasses the entire business ecosystem.
Considering the adoption of GenAI as part of a broader digital transformation strategy involves engaging stakeholders from various departments, as well as external partners such as suppliers, distributors, and customers. Only in this way can a seamless flow of information be created that results in a more agile, resilient, and responsive supply chain.
GenAI Use Cases in Supply Chain
As mentioned above, many potential use cases will emerge over the next few years, but we can already find real cases in the most pioneering companies in the industry.
Companies already using traditional AI are more advanced with generative AI and are more confident about future growth. As a result, the most common use cases are:
- Product design
- Logistics network design
- Global trade optimization
- Demand forecasting
- Supplier management
- Production yield or manufacturing quality optimization
- Risk management
- Customer service chatbots and product training in pre and post-sales
When comparing expected growth in GenAI over the next two years, the survey data shows that industry leaders are more likely to anticipate a faster progression of GenAI implementation in logistics, manufacturing, and pre/sales.
Demand forecasting
Demand forecasting is essential for supply chain management, helping companies to optimize inventory and reduce the risk of stock-outs.
This technology uses historical data, market trends, and external factors to create more accurate demand forecasts. By considering different factors at the same time, these models can identify complex patterns that traditional forecasting methods might miss.
Companies can then anticipate changes in demand and adjust production and inventory levels accordingly.
Supply chain planning
Planning driven by AI tools brings significant benefits to companies by taking into account production capacity, lead times, and demand. This process ensures a smooth and uninterrupted flow of products from manufacturers to end consumers.
This optimization of operations minimizes bottlenecks, reduces delays, and improves productivity, as well as promoting customer satisfaction and loyalty.
With optimized supply chain planning, you can reduce costs and gain a competitive advantage in the marketplace.
Inventory optimization
Avoiding out-of-stock is a major concern for companies in the industry, but so is minimizing the cost of storing too much merchandise. Generative AI helps to fine-tune inventory management by forecasting ideal stock levels based on demand trends and external factors.
This reduces overstocking and inventory and makes supply chains more agile. It identifies the most efficient distribution and warehousing methods, taking into account lead times, transportation costs, and changes in demand
Predictive maintenance
Predictive maintenance, facilitated by generative AI models, offers substantial advantages by leveraging data from machines on the shop floor that predict when equipment is likely to fail.
It also allows manufacturers to optimize maintenance schedules, minimizing downtime and costs while extending equipment life.
Fraud detection
Models can be trained to predict the likelihood of fraud occurring in the industry. By analyzing financial data, GenAI can identify intricate patterns, thus helping to detect fraudulent activity.
The use of GAN significantly improves the accuracy of fraud detection, also helping to strengthen supply chain security.
Product design
Generative AI accelerates the innovation process through its ability to generate and evaluate numerous alternative designs according to specific criteria.
This approach accelerates the design process and product quality. This can lead to a higher rate of customer satisfaction and gain competitiveness in the marketplace.
GenAI Supply Chain Management
The correct implementation of generative AI in supply chain companies involves a complex interplay of technical, organizational, and operational challenges. As such, there are a few key steps to be taken to move towards a more productive and optimized path:
- Align people and investments with strategic vision: It is important to have a cohesive vision and external support to ensure that GenAI pilots and implementations are focused on generating business value.
- Prioritize data readiness when considering use cases: the demands of this technology are highlighting the myriad complexities of enterprise data management. Therefore, data availability, quality, and privacy must be prioritized when prioritizing use cases.
- Maximize the value of GenAI by mitigating cyber and data risks: this is an emerging technology, so it also involves risks. Risks must be reduced and opportunities optimized to achieve more robust cybersecurity.
The idea of an autonomous supply chain ranges from enthusiasm to skepticism from industry leaders, but generative AI is already proving that it can be an innovative and critical element in this sector. And having an expert partner will be crucial when it comes to implementation.
At Plain Concepts, we help you design your strategy, protect your environment, choose the best solutions, close technology and data gaps, and establish rigorous oversight to achieve responsible AI. You can achieve rapid productivity gains and build the foundation for new business models based on hyper-personalization or continuous access to relevant data and information.
We have a team of experts who have been successfully applying this technology in numerous projects, ensuring the security of customers. We have been bringing AI to our clients for more than 10 years and now we propose a Framework for the adoption of GenAI:
- Unlock the potential of end-to-end generative AI.
- Accelerate your AI journey with our experts.
- Understand how your data should be structured and governed.
- Explore generative AI use cases that fit your goals.
- Create a tailored plan with realistic timelines and estimates.
- Build the patterns, processes, and teams you need.
- Deploy AI solutions to support your digital transformation.
Preparing your company to successfully adopt generative AI is the core of our framework, where we will cover 4 main pillars: strategy and governance of data and your privacy, security and compliance, reliability and sustainability, and responsible AI. This will help you avoid the risk of projects never making it to production.
Don’t wait any longer and start scaling your GenAI solutions!