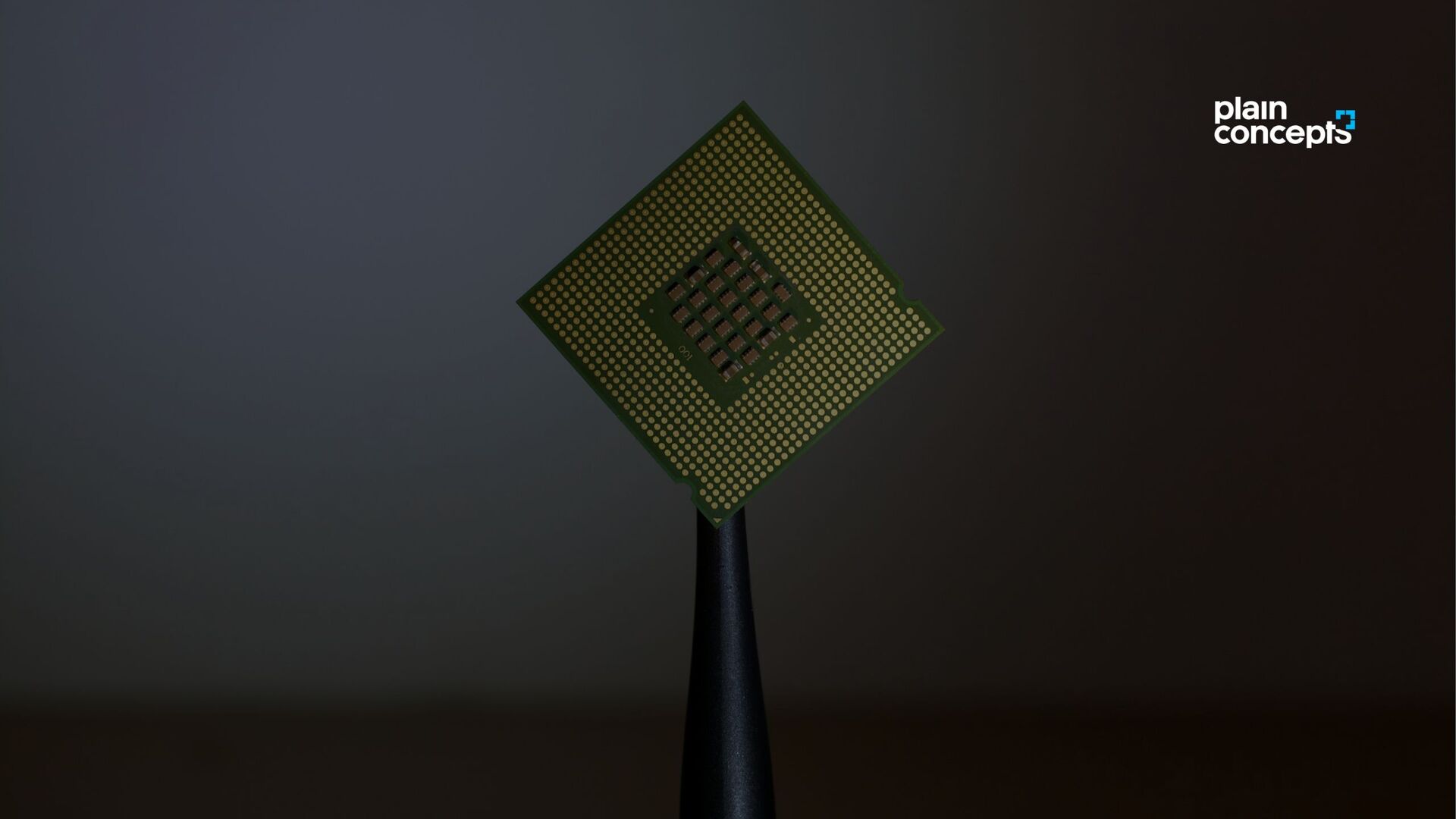
Ethics and Machine Learning: Present and Future Challenges
Machine Learning has the potential to transform society and the way we do business in many positive and meaningful ways, but it is critical to consider the ethical implications of the models and systems.
As AI systems become increasingly sophisticated and integrated into our daily lives, it is critical to address the ethical dimensions linked to their growth. We analyze their implications, challenges, and the keys to overcoming them and achieving projects based on responsible AI.
Ethics in Machine Learning
The key principles of Machine Learning ethics are based on four fundamental pillars:
- Fairness: it must be ensured that ML algorithms do not discriminate against individuals or groups based on characteristics such as race, gender, or age. Therefore, fairness is a fundamental principle in the ethics of this technology, as it seeks to eliminate bias and promote equal treatment.
- Transparency: clear and understandable explanations of how algorithms make decisions must be provided to foster accountability and trust. Transparency is therefore essential to ensure that those affected by algorithmic decisions can understand the factors that influenced those decisions. This can be achieved through a variety of means, such as providing access to the underlying code, documenting the decision-making process, and disclosing the data used to train the algorithm.
- Privacy: Privacy has become a fundamental right that must be protected through data protection and ensure that it is not misused or exploited. Responsible collection, storage and use of data and the implementation of robust security measures to prevent unauthorized access should be advocated.
- Accountability: Holding developers and users of ML systems accountable for their actions and for the negative results their systems may generate is necessary to ensure that these algorithms are used in a way that conforms to ethical principles, moral responsibility, and social values. This can be achieved through clear guidelines, standards, and oversight mechanisms.
By adhering to these key principles, the development and responsible use of ML algorithms can be promoted. In addition, trust, fairness, and accountability in the implementation of these systems can be fostered, thus benefiting individuals, society, and many sectors.
Machine Learning Challenges
AI systems possess the ability to learn and make decisions autonomously, mimicking human intelligence, but this progress raises many questions about the ethical issues associated with the use of this technology.
One of the main ethical issues related to AI is privacy and data protection. AI systems handle large amounts of personal data, so there is a great deal of concern surrounding the methods that AI algorithms use to collect, store, and use this data.
On the other hand, the unintended use of biased or discriminatory data and algorithms further complicates the issue. Potential ethical dilemmas in the workplace or economic inequality are other major concerns.
Algorithmic bias
This is one of the most important challenges facing ML and is the aspect that should ensure that people are treated equally when making decisions. Algorithms should not give preferential or discriminatory treatment to a specific group of people.
Ethical issues are not only limited to bias but also include the possibility that a specific group of people may not be able to access the services of the systems. For example, persons with disabilities or language limitations should not have difficulty using AI-ML services.
Data privacy
The demand for the protection of individual and corporate information has grown tremendously, especially over the last decade, resulting in regulations such as the GDPR, HIPAA, or CCPA.
These regulations dictate how service providers must store and process data collected from users based on intent.
In addition to data protection, organizations must also protect the different processes that handle the collected data, such as preprocessing, version control, analysis, reuse, and storage.
Transparency of algorithms
Ensuring that algorithms are not opaque is another challenge for the trustworthiness of this technology, as it affects the accountability of AI and ML systems for the decisions they make and the logic behind them. So it is a combination of governance and explainability.
It is important to tackle the failure to provide explanations behind decisions, including traceability and low-level or data-specific interpretations, which generate distrust.
Human security
AI and ML models have the potential for exploitation, as what may have started out as a positive use can be modified to pursue malicious goals. Therefore, models must be reliable, so that their functions are reproducible under conditions similar to those under which they were developed and tested.
In addition, robust systems must have the ability to cope with unpredictable situations, as unknown scenarios may expose users to risks such as identity exposure or loss of credentials.
Trustworthy AI in companies
Ranking Digital Rights conducted a study analyzing users’ trust in large technology companies based on their algorithm-based data curation practices, which showed a discouraging picture.
While companies have accepted that AI will improve work processes and expected returns, they have also realized how important it is to invest in AI research.
One of the reasons for this is the benefits a company can achieve when it relies on reliable AI:
- Increased user participation: there is a strong correlation between data ethics and user participation, as users will be willing to share their information if they believe they will be protected from unethical use.
- Increased accessibility and reliability: recognizing risks and developing procedures to manage them will result in a more accessible and reliable product.
- Healthier economy: socio-economic and political impacts are a recurring theme in debates about unethical AI, so adopting good practices helps companies promote a thriving economic environment and steer clear of legal conflicts.
- Conscious computing: Algorithms that care for the environment and people can attract monetary incentives such as carbon points.
Achieving reliable AI
A system that preserves ethics is a big step toward using technology for human welfare. Some of the steps to achieve reliable AI include:
- Encourage participation: Knowing the range of intended stakeholders and their concerns will enable a holistic approach, broadening the view of the product from the time of design.
- Data minimization during collection: this is a good practice to ensure privacy compliance when collecting user data. This will allow sufficient data to be collected for the purpose of the use case, but avoid going overboard if there is no specific purpose.
- Ensure accessibility: a user-friendly interface and affordable access mechanisms should be achieved.
- Periodic evaluations: technology is constantly changing, so it should be regularly audited for fairness, transparency, and robustness.
- Monitoring models: This will be very important to detect violations and data-related problems, as well as to be alerted to potential problems.
- Audits of results: Fairness metrics, including measurement of bias, should be taken into account during design. In addition, robustness metrics and the results of explainability reports should drive product development.
Responsible AI needs to be embedded throughout the organizational structure, but improving awareness and measures of responsible AI requires changes in operations and culture. Therefore, the right choice is to rely on a technology partner who understands the importance of this type of business approach.
This approach facilitates faster innovation and minimizes risk, so companies that ride the next wave of regulation by following responsible business practices will be able to satisfy legislators, shareholders, and customers with cutting-edge, secure products and services.
At Plain Concepts we have been recognized as Microsoft Partner of the Year 2023 in Responsible AI thanks to our focus on education and awareness of the ethical aspects and responsible use of AI-based on two main pillars: internal training and communication with our customers.
Internally, we provide regular training to our employees on the ethical aspects of AI, promoting a thorough understanding of the challenges and best practices in this area. With customers, we focus on education and advice, providing them with clear and accurate information on ethical issues and responsible use, guiding them in making ethical decisions in their projects.
We provide you with different tools to better understand and know how the different algorithms developed respond. We adapt to new legislative changes and to your needs in order to embark on a joint path toward efficiency and responsibility. If you want to know how, do not hesitate to contact us and our experts will be able to advise you.