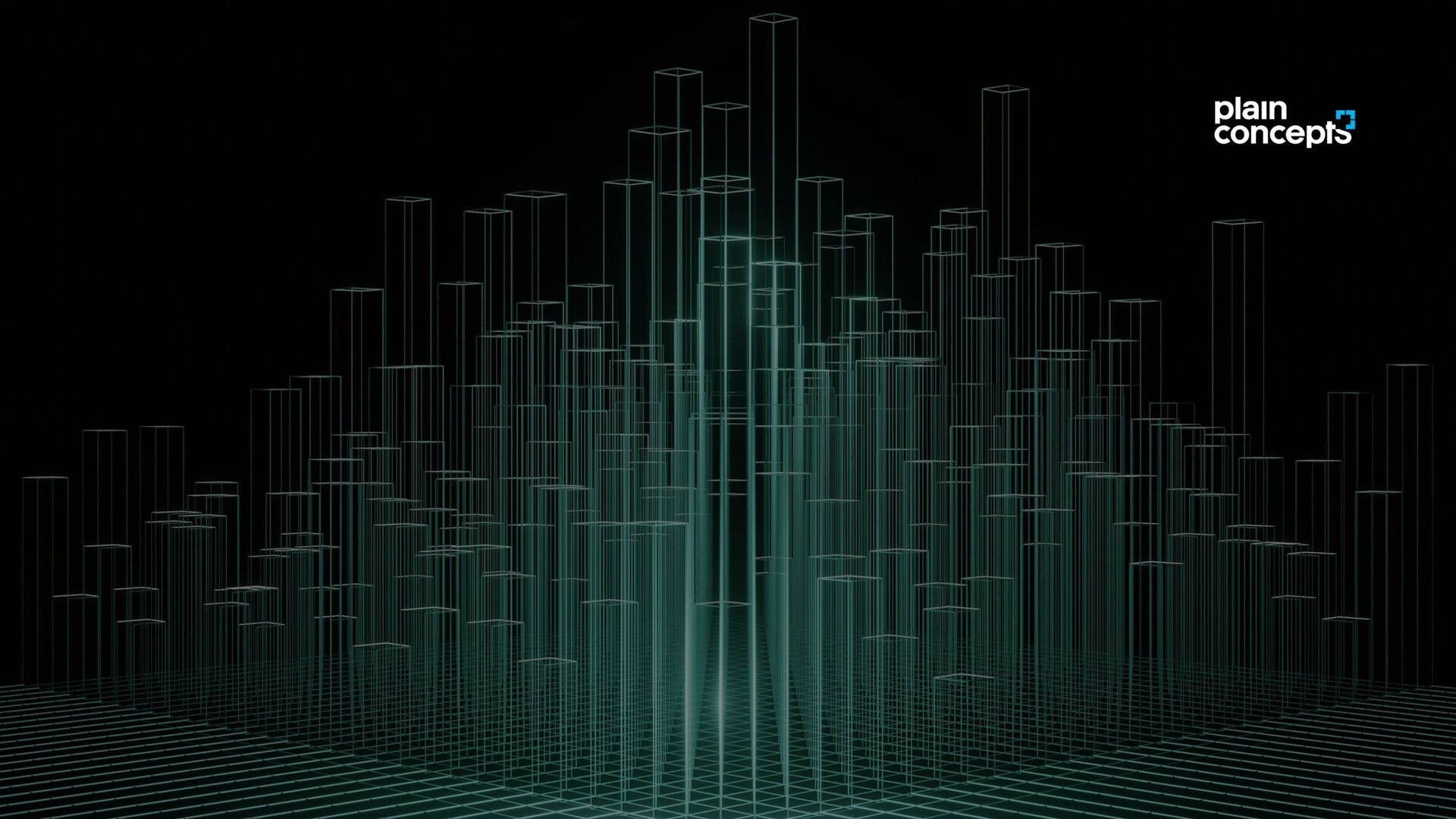
Digital twins and generative AI: the perfect duo
Generative AI and digital twins are increasingly becoming two technologies that are less unknown to businesses in any industry. Many organizations implement them separately, thanks to their huge potential to create many use cases.
However, few companies have been able to see the synergies they can provide by combining the two, such as reducing costs, accelerating the implementation of the other, and providing greater value than separately. We explain the advantages and use cases they provide when combined.
Digital Twin Generative AI
Digital twins are a powerful tool that helps businesses improve data-driven decision-making, simulating strategies, and optimizing behaviors.
Creating scenarios of real-world situations and outcomes provides insights that serve as early warnings, predicting events and the likelihood of problems occurring. They are also the perfect digital tool for testing and trialing options without compromising real assets, improving efficiency and time to market.
For its part, generative AI is a technology that has created numerous use cases since its recent launch and, like the digital twins, it is revolutionizing business processes and activities. As such, the impact on productivity and economic potential is multiplying with each one.
In fact, according to McKinsey, 75% of large enterprises are investing in digital twins to scale AI solutions. Each technology has huge potential on its own, but together they could unlock trillions in total economic value.
As the technological world is constantly changing, we are at a very important time when businesses can start using GenAI to drive predictive analytics, improve AI models, and help build smarter digital twins.
By using this technology strategically, users are moving towards smarter operations, bridging the gap between the digital and physical realms, giving more control and a better understanding of business processes.
Benefits of combining generative AI and digital twins
Combining data with problem-solving in innovative ways can improve efficiency as well as sustainability. Some of the benefits of bringing these two technologies together include:
- With AI, engineering becomes a predictive field that improves forecasting and accuracy.
- AI models enable refined resource allocation.
- It revolutionizes asset tracking and maintenance, elevating operational readiness.
- It can translate thousands of pieces of data into actionable strategies, providing insight and foresight in decision-making.
- It is a cultural shift towards intelligent efficiency and progression.
Increased value by joining forces
Creating a digital twin, especially for highly specialized applications, can be time-consuming and resource-intensive. This effort often involves designing and developing new models, a process that can take months and incur many costs.
LLMs can create code for the digital twin, speeding up the development process and increasing efficiency. This ability to generate such output suggests that these models can be used to create a generalized (universal and fundamental) digital twin solution that facilitates the design and serves as a starting point for developers in a variety of projects and industries.
The architecture of a digital twin can be represented as nodes and edges in a time series graph, allowing graph-based LLMs to create a basic model of a twin, the design of which can then be developed and adapted to various scenarios.
These twins thrive on large volumes of data, which can be difficult to manage. To address this, LLMs offer advanced ‘embedding’ capabilities, meaning they can significantly compress data and retain essential information at the same time. This enables the efficient transfer and processing of data so that digital twins can analyze it, identify patterns or anomalies, and inform better decision-making and predictive maintenance strategies.
In addition, AI tools can complement the training datasets used by digital twins by creating synthetic data or communicating through natural language.
In fact, generative AI can learn and extend the scope of indications and results, generating ‘what-if’ simulations run by digital twins, where users can make adjustments and thus achieve predictive models.
GenAI and Digital Twins Use Cases
The collaborative link between AI and digital twins is critical to developing systems that mirror their physical counterparts, as well as being predictive and adaptive. With the integration of both technologies, a dynamic model can be achieved that learns, evolves, and provides actionable intelligence that informs real-world decisions.
The development of digital twin capabilities has been meteoric over the last few years, and AI and ML algorithms have reinforced these capabilities, broadening the horizons of both in numerous use cases, such as real-time monitoring, predictive maintenance, scenario planning, etc. Here are some of the most important ones.
Generative Digital Twins
A generative digital twin agent could take care of the integration through APIs of the model and the data. Acting as a ‘generator’, it significantly accelerates the implementation phase of digital twins, offering organizations a simpler approach to adopting this technology.
This use case can have a major impact on the way industries employ digital twins to improve their operational efficiency.
Asset performance management
Large-scale models can be created based on time series data and correlated with work orders, event prediction, health scores, criticality indices, or other unstructured data for anomaly detection.
Using AI models to create digital twins of assets helps to make all historical information accessible for current and future operations.
Generative intelligence
Generative intelligence is also transforming digital twins from static to dynamic models capable of providing expert guidance and decision support.
By bringing them together, digital twins evolve into highly intelligent entities, that assist teams by offering data replication, strategic advice, and predictive analytics.
The integration of advanced cognitive functions in digital twins sets a new standard in how we interact with and leverage technology, ensuring that these systems are intelligent and advisory in applications from industrial machinery maintenance to urban infrastructure management, etc.
Multi-agent generative systems
Intelligent software agents with specialized knowledge can be trained using advanced linguistic models, allowing them human-like interaction capabilities.
They can function as a generative multi-agent system in which each agent collaborates and organizes itself autonomously. They are used to perceive, memorize, reflect, and execute actions aligned with company-specific operational guidelines.
This integration of intelligent agents and digital twins streamlines workflow, ensuring the efficiency and adaptability of operations.
Visual information
By creating a model of various asset classes and leveraging large-scale visual images and adapting them to the configuration of each case, neural network architectures can be used.
This can be used to scale the use of AI to identify anomalies and damage to service assets instead of manually reviewing the image.
Making the most of generative AI and digital twins
Leaving aside the advantages of these technologies, the use of generative AI can lead to potential inaccuracies or biases, so it is very important to ensure that the tools are optimized for user privacy and regulated data.
During the development process, it is important to carefully consider the assumptions and simplifications of the models to ensure that the results are contextualized. Continuous monitoring is also essential to ensure that models do not produce misleading results.
This can be avoided by ensuring that digital twins and AI are built on a high-volume, high-quality database. Therefore, before creating a digital twin, it is very important to be clear about the business use case and to have secure, quality data.
Generative AI can present ethical challenges for businesses, which is why it is so important to have a technology partner to help maintain a culture of continuous learning and development, as well as provide the necessary tools to protect the data and ensure its ethics.
At Plain Concepts we have years of experience in developing cutting-edge technologies, always with a focus on security and creating real value. Contact us
!