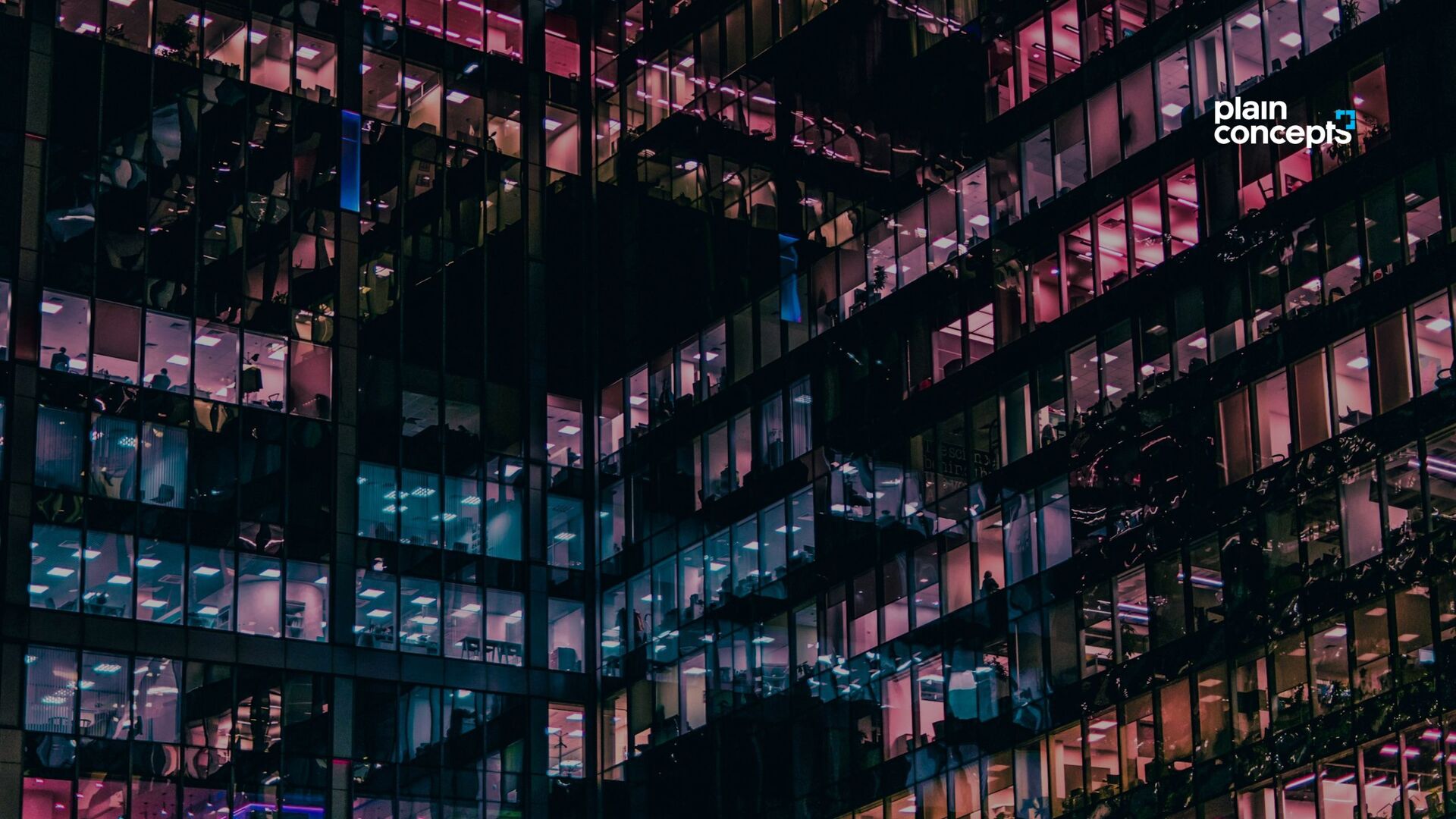
Keys to building a data architecture that drives innovation in your business
- What is a Data Architecture?
- Benefits of a Modern Data Architecture
- How to build an innovative Data Architecture?
- From on-premises data platforms to cloud platforms
- From batch processing to real-time
- From pre-integrated commercial solutions to modular platforms
- From point-to-point to decoupled access points
- From an enterprise data warehouse to a domain-driven architecture
- From rigid data models to flexible and extensible models
- How can Data Architecture drive Innovation?
Over the past few years, organizations have had to move quickly to implement new technologies that leverage the power of data while trying to upgrade legacy infrastructures to deliver more innovative services.
These additions and customer demands have increased the complexity of data architectures, which can greatly hamper the ability to maintain existing infrastructures and ensure the smooth operation of new ones.
Data is the fuel that drives business. Do you belong to the high percentage of companies that struggle to implement a successful data strategy? If the answer is yes, here are the keys and steps to change that and achieve a data architecture that can meet today’s needs for speed, flexibility, and innovation.
What is a Data Architecture?
A data architecture is the way in which data is managed, from collection to transformation, distribution, and consumption.
It is responsible for establishing the model for data and the way it flows through storage systems. As such, its design must be driven by the company’s business requirements and to meet specific business needs.
As new sources of data emerge through new technologies, a good architecture ensures that the data collected is manageable and useful, as well as avoiding the storage of useless data, improving data cleansing and quality.
These modern platforms leverage cloud platforms to manage and process data, making their scalability to quickly complete processing tasks and ensure relevant data is available to improve the quality of AI applications.
Benefits of a Modern Data Architecture
A good data architecture streamlines the way we manage and design data infrastructures.
The characteristics that differentiate modern data architectures from legacy systems are as follows.
Scalability
Traditional architectures cannot cope with growing volumes of data, so they must scale.
Virtualization has raised the level of convenience and elasticity for organizations, allowing them to scale performance and manage rooms, servers, nodes, and containers.
This provides convenience, speed to upgrade servers, and disaster recovery.
Therefore, scalability is key to a modern data architecture, allowing it to adapt as business demands change.
Automation
With scalability come new maintenance and management challenges. The volume of data that companies handle is too large, and tasks need to be automated so that databases can act independently.
This can be applied to identify and correct errors and create reports or data structures, among many other applications.
This is where the DataOps methodology comes in, helping to improve communication, integration, and automation of data flows between data managers and data consumers in an organization.
Cost savings
Such large volumes of data in on-premises systems require a large outlay of costs to get up and running. Leaping to a cloud platform is also a large upfront investment, but one that will pay off handsomely, thanks to its huge benefits.
Modern data architectures streamline costs and eliminate unnecessary fees, all while keeping the business running smoothly.
Data quality
Data quality is a fundamental pillar for businesses, as poor data quality has a very high financial impact.
Modern architectures maintain data quality dimensions and periodically check for inaccuracies, anomalies, or inconsistencies.
The cloud plays an important role here, as it allows much of this process to be automated, ensuring quality from the outset.
Simplicity
As with almost everything in life, simplicity is the best, and this also applies to data architecture.
The best option is the one that best suits the needs of each case without exceeding the possibilities of each company and opting for the model that brings us the maximum value.
How to build an innovative Data Architecture?
While it is possible to implement some changes and leave the core structure intact, most companies need to restructure the existing data infrastructure, which includes legacy and new technologies to be incorporated.
According to McKinsey, companies are implementing six fundamental changes to their data architectures to deliver new capabilities faster and simplify existing approaches. These are summarised below.
From on-premises data platforms to cloud platforms
The cloud is one of the pillars underpinning modern data architecture because, as mentioned above, it makes it easy to scale AI tools and capabilities for greater competitive advantage rapidly.
This is why leading cloud providers have completely changed the way organizations procure, deploy, and run their infrastructure, data, and applications.
There are two types:
- Serverless data platforms, which allow organizations to build and operate data-centric applications without the expertise, speeding deployment and reducing operational costs.
- Containerized data solutions, supported by Kubernetes, allow enterprises to decouple and automate the deployment of data warehousing systems. It is intended for more complicated, but highly scalable configurations.
From batch processing to real-time
The costs of real-time data transmission capabilities have fallen dramatically in recent years, which has democratized their use.
These technologies are responsible for creating new business applications, such as subscription mechanisms, taxi driver location information, prediction of infrastructure problems, analysis of customer behavior, etc.
From pre-integrated commercial solutions to modular platforms
When it comes to scaling applications, companies often encounter obstacles with legacy data. This is why a modular data architecture can be the best solution.
For this reason, many utilities are turning to this approach to quickly deliver new data-intensive digital services and connect cloud-based applications at scale.
As such, data pipelining and API-based interfaces simplify integration between disparate tools and platforms by shielding data teams from the complexity of different layers, speeding time to market, and reducing the possibility of causing new problems.
From point-to-point to decoupled access points
Exposing data via APIs can ensure that direct access to view and modify data is limited and secure and provides faster and more up-to-date access.
This means that data is easily reused across teams, speeding up access and smoother collaboration.
For example, an internal ‘data mart’ for employees can be created via APIs to simplify and standardize access to core data assets rather than relying on proprietary interfaces.
From an enterprise data warehouse to a domain-driven architecture
Many companies have moved from a central data lake to domain-driven designs that can be customized to the business objective and thus improve the time to market for new products and services.
While data sets can still reside on the same platform, this approach tasks the owners of each departmental domain with organizing their data sets in an easily consumable format.
It therefore requires a carefully considered balance to avoid becoming fragmented or inefficient. However, it significantly reduces the time spent building new models within the data lake, and can be a simpler and more effective option.
From rigid data models to flexible and extensible models
It is common for pre-defined data models from software vendors to be built on standardized schemas with rigid database tables and data elements to minimize redundancy. This approach is useful for use cases focused on reporting and regulation, but it involves investing in long professional development cycles when new elements or data sources are to be incorporated.
Therefore, a more flexible, de-normalized model provides a powerful competitive advantage when exploring data or supporting advanced analytics. It brings numerous benefits, such as agile exploration, reduced complexity, better storage of structured and unstructured data, etc.
How can Data Architecture drive Innovation?
Investments in building enhanced capabilities can be a high initial cost for companies, which is why it is so important to have a clear strategic plan to make the best decisions and prioritise those changes that will directly affect business objectives and not invest more than necessary.
When this is done well, the return on investment can be very significant, as well as bringing numerous benefits, such as productivity improvements, reduction of regulatory and operational risk, delivery of capabilities…
Some of the keys to implementing new technologies in our architecture include:
- Apply an agile mindset to test and learn from the architecture, with the goal of experimenting with different components and concepts.
- Establish data ‘tribes,’ where different teams work together to implement standard data and feature engineering processes to support the development of model-ready datasets.
- Invest in DataOps to accelerate the design, development, and implementation of new components.
- Create a data-driven culture and link it to the company’s business objectives.
At Plain Concepts, we guide you through the entire data lifecycle to maximize your business’s value. We aim to approach the challenge of digital and data strategy from a business prism with which to obtain benefits thanks to a structured framework according to your needs.
With this approach, we define the necessary strategy through a process of immersion, maturity, and consolidation, working on the generation of short-term benefits that give credibility to this strategy.
Based on the degree of maturity, we propose an evolutionary roadmap to leverage the data. To this end, we have developed a specific framework to better define the path to becoming a digital company, addressing strategy, operating model, organization, and technology.
If you want to start getting the most out of your data, we will be your best ally!