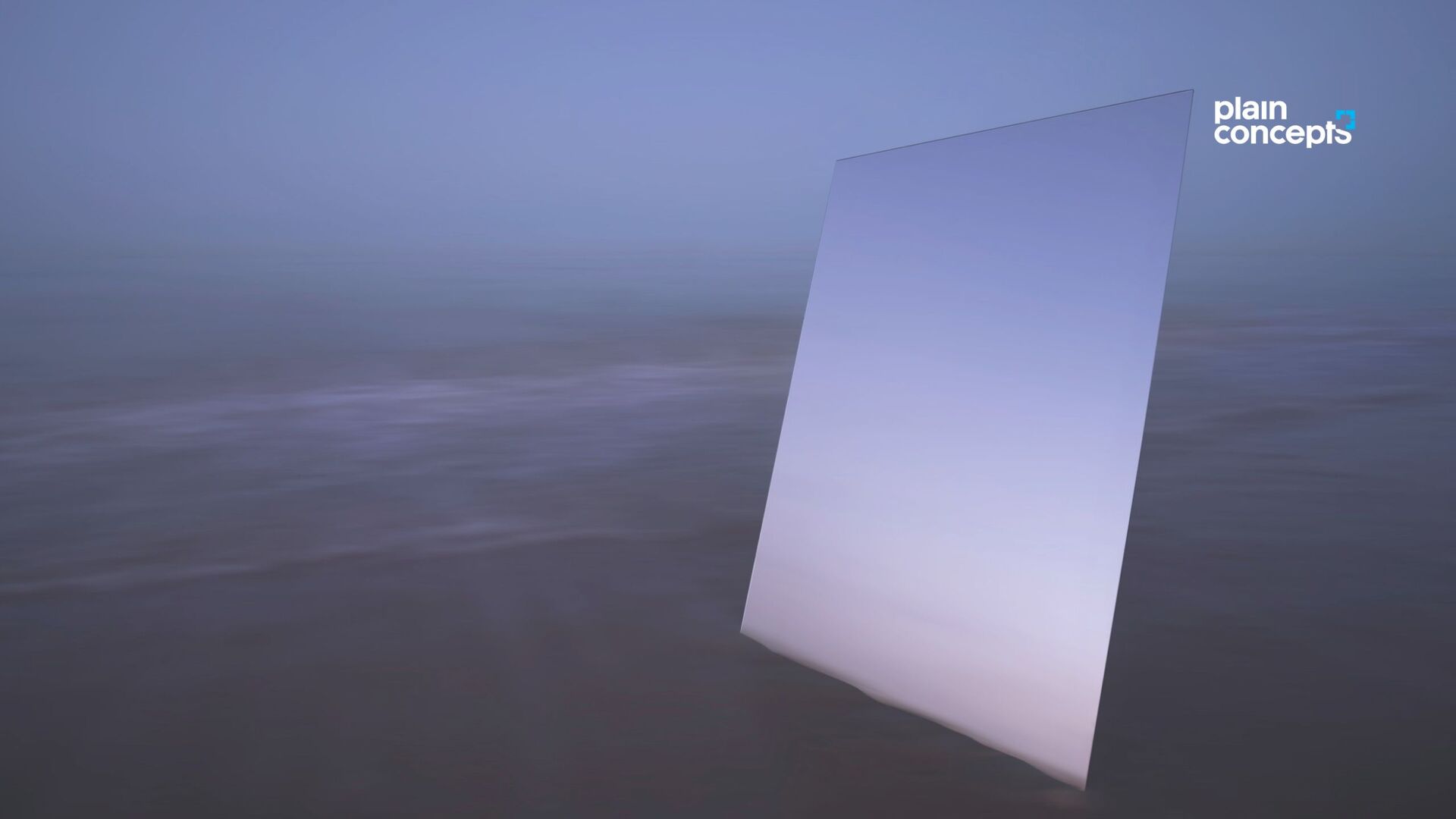
AI Transparency: Fundamental pillar for ethical and safe AI
It is already undisputable that artificial intelligence is rapidly transforming the business world as it becomes increasingly integrated into the fabric of organizations and users’ lives.
However, its rapid evolution also opens a door to some risks as organizations wrestle with challenges around implementing this technology responsibly to minimize risk.
One of the pillars of responsible AI is transparency, and companies must take steps now to ensure that models are unbiased, ethical, and fair. We review what responsible AI consists of, its challenges, and the keys to applying it in your business.
What is AI Transparency?
We can define AI transparency as the ability to understand how AI systems work, encompassing concepts such as explainability, governance, and accountability of AI.
Ideally, this visibility of AI systems should be integrated into every phase and facet of the model, from ideation, understanding, development, to data categorization, fault detection and frequency, to communication between developers, users, and stakeholders.
With the advent of generative AI and the evolution of Machine Learning models, the many facets of AI transparency have come to the fore, but there has also been growing concern about the more powerful models, which are more difficult to understand.
Why is transparency in artificial intelligence important?
Like any data-driven tool, AI algorithms depend on the data quality used to train the model. These algorithms are subject to biases, an inherent risk in their use. This is why transparency is essential to ensure the confidence of users, regulators, and those affected by algorithmic decision making.
AI transparency is becoming a very important discipline in the AI field because of the need for trust, auditability, compliance, and explainability. Without transparency, we can run the risk of creating AI models that may inadvertently perpetuate harmful biases, make inscrutable decisions, or lead to dangerous outcomes in high-risk applications.
Indeed, with the advent of generative AI, it has put even more focus on the importance of transparency and increased pressure on companies to improve their governance around the unstructured data LLMs work with.
Their complex interactions within the huge neural networks used to create these GenAI models make decision-making processes less transparent, as these can exhibit unexpected behaviors or capabilities. This raises new challenges on how to ensure the transparency of unanticipated functions.
AI Transparency Challenges
AI transparency is a work in progress as the industry discovers new problems and better processes to mitigate them.
Transparent AI practices offer numerous benefits, but they also raise security and privacy concerns. For example, the more information provided about the inner workings of an AI project, the easier it is for digital criminals to find and exploit vulnerabilities.
Another challenge is the trade-off between transparency and intellectual property protection. But it is not the only one, as we also face the barrier of giving clear explanations of complex programs and Machine Learning algorithms to non-experts.
On the other hand, there are currently no global transparency standards for AI either, which is yet another challenge to add to the list.
Transparency vs. Explainability vs. Interpretability vs. Data Governance
As mentioned above, AI transparency is built through numerous processes aimed at ensuring that all stakeholders have a clear understanding of how an artificial intelligence system works, including how it makes decisions and processes data.
- Explainability is the ability to describe how the model algorithm arrives at its decisions in a way that is understandable to non-experts.
- Interpretability focuses on the inner workings of the model, with the goal of understanding how its specific inputs led to the model’s output.
- Data governance provides information on the quality and appropriateness of the data used for training and inference in algorithmic decision making.
Although the first two are crucial to achieving AI transparency, they are not all-encompassing, as they also involve being transparent about data handling, model limitations, potential biases, and the context of its use.
For all these reasons, data transparency is fundamental to AI transparency, as it directly affects the reliability, fairness, and accountability of AI systems.
To ensure this, the origin of data sources, how they were collected, and any preprocessing processes they have undergone must be clearly documented. Thus, these tools will enable organizations to trace data flows from source to outcome, ensuring that each step of the AI decision-making process is auditable and explainable.
Best practices for implementing AI transparency
As we have discussed throughout the article, AI is transforming and will transform the lives of businesses, people, and industries globally. In fact, used correctly, it can be a powerful tool to address global challenges such as climate change or social inequalities.
The transparency of artificial intelligence is multi-faceted, so teams must identify and examine each of the potential issues hindering it. Decision-makers must consider the broad spectrum of transparency issues:
- Process transparency audits during development and implementation.
- System transparency provides visibility into AI usage.
- Data transparency helps to have visibility into the data that is used to train AI systems.
- Consent transparency informs users how their data might be used in AI systems.
- Model transparency reveals how AI systems work, possibly by explaining decision-making processes or making algorithms open source.
To this end, the full potential of this practice must be addressed with a focus on transparency when implementing AI. To this end, a few steps can be taken to promote good practices:
- Develop a framework that supports the responsible use of AI: Frameworks help ensure that AI is used responsibly, safely, and fairly, making it easier for users to have more confidence in their AI models.
- Interact proactively from the start: Keeping all parties involved from the beginning will be crucial when making decisions, as well as overseeing and monitoring aspects to ensure that models work well.
- Consider diversity and avoid bias: A diverse development team is critical to mitigate the risk of bias, as well as helping AI tools meet the needs and expectations of organizations.
- Apply governance principles: Companies must define objectives, risks and carefully monitor their AI strategy, as well as ensure that AI is used and implemented responsibly and that users are properly trained. Applying governance principles will ensure that models can be properly assessed and safeguards put in place.
- Integrate security barriers: these are fundamental to the responsible use of models. They can be viewed as algorithmic safeguards or a set of filters and rules designed to ensure that AI systems operate ethically and legally.
At Plain Concepts, we can help you clarify all your doubts and shape a strategy that creates real value for your business. We provide you with different tools to better understand and know how the different developed algorithms respond. We adapt to new legislative changes and your needs to embark on a path together towards efficiency and responsibility.
We will design your strategy together, so that you have a protected environment, choose the best solutions, close technology and data gaps, and establish rigorous oversight for responsible AI. You can achieve rapid productivity gains and build the foundation for new business models based on hyper-personalization or continuous access to relevant data and information.
We have a team of experts who have been successfully applying this technology in numerous projects, ensuring the security of customers. We have been bringing AI to our clients for more than 10 years, and now we propose an AI Adoption Framework:
- Unlock the potential of end-to-end generative AI.
- Accelerate your AI journey with our experts.
- Understand how your data should be structured and governed.
- Explore generative AI use cases that fit your goals.
- Create a tailored plan with realistic timelines and estimates.
- Build the patterns, processes, and teams you need.
- Deploy AI solutions to support your digital transformation.
Start your journey towards a more transparent AI now!